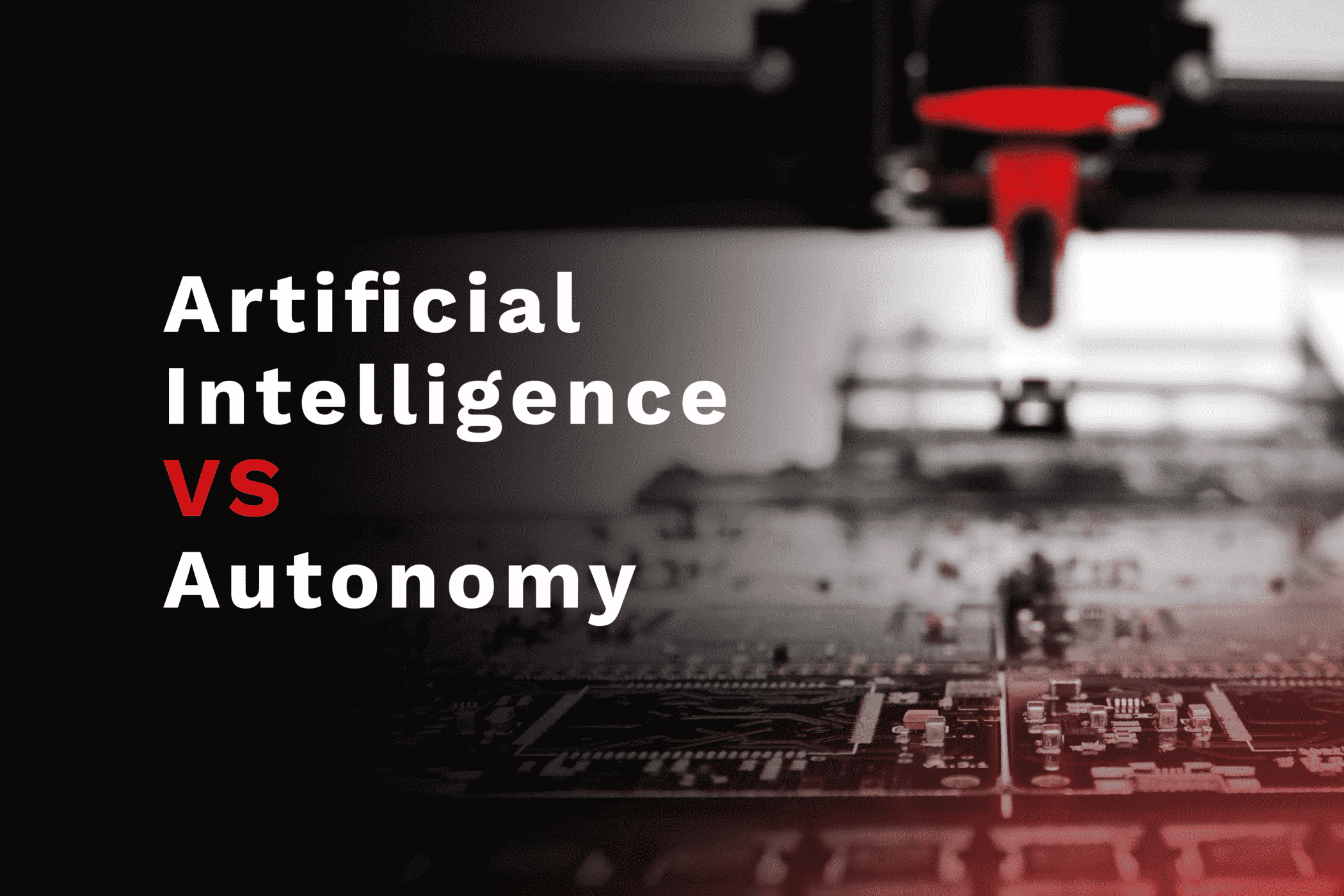
Artificial Intelligence vs. Autonomy: 2 Different Concepts That Are Key to The Future of Mobile Robotics
Autonomy and artificial intelligence (AI) are often used interchangeably in conversation and in the media. These two concepts, however, are quite different in practice. Understanding the difference between AI and autonomy will help your company to make the most practical choices for greater productivity now and in the future. At Inertial Sense, we specialize in integrating AI, machine learning and autonomous systems to create the right solutions for our clients’ autonomous robotic systems needs.
Artificial Intelligence vs. Autonomy
Artificial intelligence applications and autonomy are both valuable tools in the industrial environment. These technologies can be used independently or can work together to achieve the desired results. Here’s an easy way to breakdown the differences between the two: autonomous robotics = task completion and AI = problem-solving.
- Autonomous robotics systems are designed for use in predictable environments to complete tasks within a specific, usually pre-planned, environment. Sensors are of critical importance in providing robots with detailed and accurate information about their location within the domain. Autonomous robotics systems rely on these sensors to navigate their environments and to perform their tasks quickly and effectively. Autonomous devices and systems can be powered by conventional software or by AI systems that allow them to learn and adapt as they operate.
- Artificial intelligence is defined by Yale University as “building systems that can solve complex tasks in ways that would traditionally need human intelligence.” This typically involves machine learning technologies and the use of highly advanced sensors to collect information about the environment and to allow the system to react appropriately to external stimuli.
We will go in-depth on the differences between AI and autonomous robotics to help you understand how they work together in the industrial and commercial settings. In general, however, it is helpful to consider AI as a tool and technique that can be used to produce autonomous robotics an
How Autonomous Robotics Systems Work
According to an article published in 2020 in the Proceedings of the National Academy of Sciences of the United States of America, autonomous systems are already filling in for people in a wide range of tasks. The future will see even greater deployments of these systems in the medical, industrial, agricultural and manufacturing sectors of the economy.
Autonomous systems can be categorized according to the amount of human interaction required for them to operate:
- Direct-interaction robotics systems are almost completely controlled by an operator. This process is also referred to as teleoperation and requires input from humans to make each change in position, attitude and status (think excavators, cranes, UAV drones and etc.).
- Operator-assisted robotics applications require the assistance of a human operator in certain high-end tasks or as part of the overall governance of the system. The machine can perform certain activities and make certain choices. For the most part, however, these systems require supervisory input from a human to choose tasks or to complete them successfully.
- Fully autonomous systems can operate without the assistance of an operator for prolonged periods of time. AI and machine learning techniques are often critical to the success of these types of systems. These fully autonomous systems are ideal for use in remote areas where direct supervision might be delayed or impossible.
By considering AI and machine learning techniques as tools that are used to achieve full or partial autonomy for robotics systems, engineers and industrial management teams can make the most practical use of these systems in real-world applications.
The Principles of Autonomous Systems
Control is critical to the proper function of robotics systems. This involves three key stages:
- Perception control is the collection of information from the environment around the robot through various sensors and the combining of that data through sensor fusion.
- Processing control takes the data from perception and weeds out extraneous or irrelevant information to allow the system to focus on the important details and information about its environment.
- Action control consists of the mechanical activities that are needed to perform necessary tasks.
AI and machine learning can be used at each of these stages to ensure the best and most efficient solutions for the tasks to which the robotics system has been assigned.
At Inertial Sense, we offer expert solutions for autonomous navigation in the industrial and commercial environments. Our precision sensors and high-end sensor fusion are designed to provide perception, processing, and action controls needed to manage navigation and to allow your robotics systems to manage dull, dangerous and dirty jobs more effectively. To learn more about our lineup of solutions for autonomy in the robotics field, give us a call today at 1-801-691-7342. The Inertial Sense team will be happy to create a customized solution precisely right for your company’s needs.