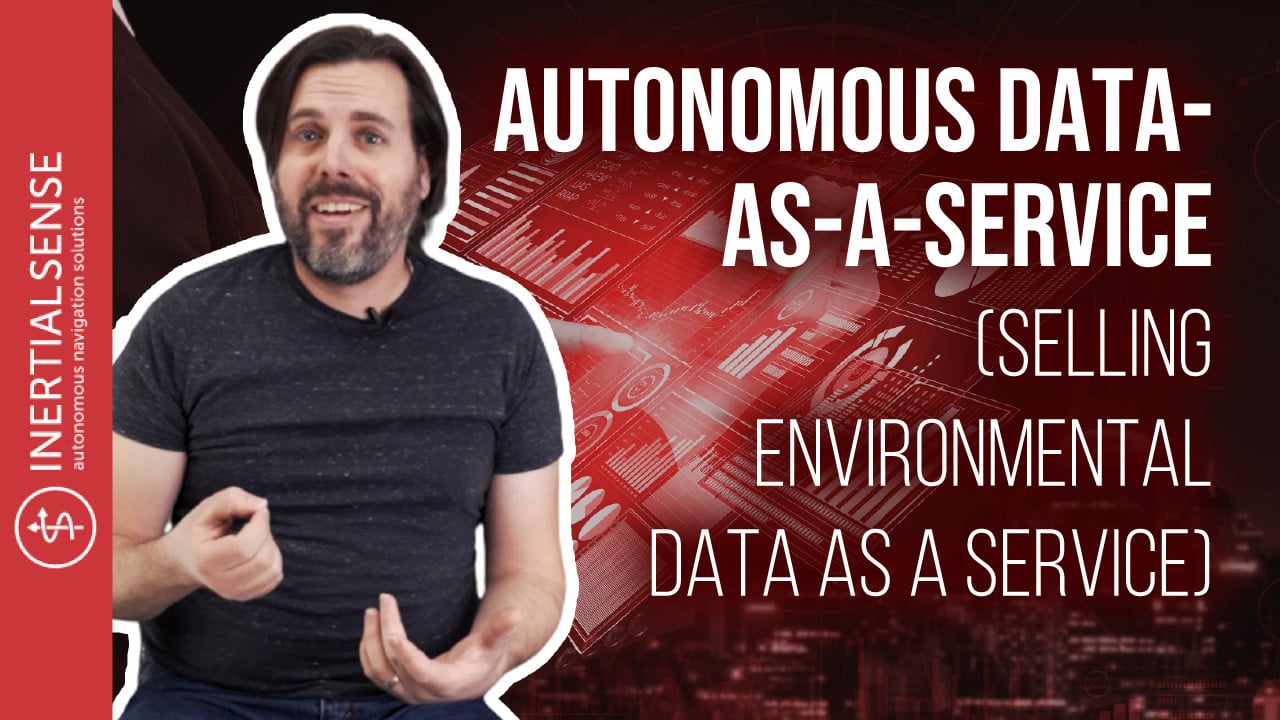
Autonomous Data-As-A-Service (Selling Environmental Data As A Service)
Have you ever wondered how technology learns and grows? Watch the video below as Joshua shares his level of expertise with autonomous data as a service and learn more about different autonomous data as a service examples.
Why is autonomous data as a service relevant to the field of robotics? Having more data transmitted to robotics allows for more data to be collected. With the combination of raw data and sensor inputs, it creates a fully developed picture.
Some more data as a service examples include: Utilizing different machine learning algorithms, robotics adapt and produce what are called features.
A feature could be something as simple as how to recognize a tree. The more trees we see, the more robust that feature becomes.
This can lead to other features like the detection of a person. Certain behaviors of what to do based on surroundings or even weather become a self-learning feature that continues to develop.
When combined with the vSlam algorithm, we can recognize and avoid or understand objects around us. The more we see of these objects or variations of objects, the more precise the algorithm becomes. The vSlam algorithm allows the recognition of an object the more it’s associated or seen. So those are a few data as a service examples that you can sell.
That said. With the right models and data, we can get accurate representation and start detecting things much easier.
Learn More:
Where is Autonomous Navigation Going in the Next 5 Years?
How Is The Field Surrounding Autonomous Robotics Advancing?
What Does The Autonomous Robotic Landscape Look Like?
Video Transcript
The more robots we have and that are tied into the Luna autonomy platform, then the more data we get. The more data we get, the more we can do.
There’s several things, one the raw data itself, which is all these different sensor inputs. That provides great things to look at.
But then, you start applying those. So there’s lots of different things that you can do. You can combine different data sources to get new results. You can run different machine learning algorithms, whether it is detecting, you know, new things with a lot of data, and the right algorithm that you start bringing out more and more what are called features.
And a feature could be something like how to recognize a tree. And the more trees that we see, the more robust that feature can become. And just as a simple example.
So, one, we can expose all the raw sensor data. Two, we can then give this really robust feature detection of a tree. And then we can give a really robust feature detection of a person. We can have certain behaviors of what’s best to do when it’s cloudy and we start losing some GPS signal or go underneath a tree.
So, the more data we have, the more things we can do with it. And as we collaborate with others and combine this data, there’s just more and more new things that come out of that.
So one of the things that we want to be able to do as we have more data, we can create more and more value of that data. With this algorithm, we use like vSlam, we’re able to recognize and avoid or understand objects around us. And the more we see of those objects or more variations of those objects, the more and more valuable, or the more precise the algorithms become, and the more valuable the data that drives those algorithms and those models become.
Like for example, if I, you know I look around and I recognize a chair, I look outside and I can see a tree, a bush is a different thing. If I see something new, even to my brain, I need to teach it, ok what is that. I need to associate it with something else. Is it close to that or something brand new? Our vSlam algorithm are doing the exact same thing
The more chairs I see or the more trees I see, the more I’m able to recognize chairs and trees in general. And that’s a little bit harder to do for computers. But with a lot of data and the right models, then we can get really accurate representation and start detecting these things easier.